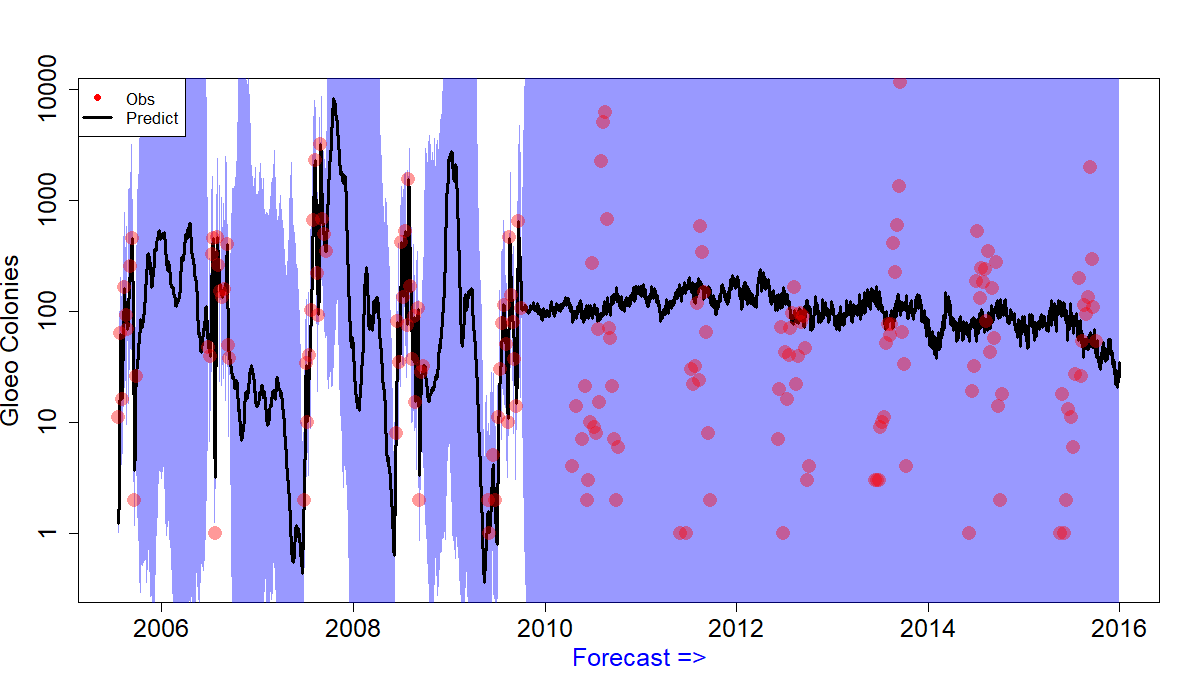
Wouldn’t it be powerful if we could predict ecological events — such as harmful algal blooms or rising risk of tick-borne disease — with the same accuracy that we can forecast next week’s weather?
Shannon LaDeau is working with quantitative and disciplinary methods to advance the field of ecological forecasting. Traditionally, ecologists fit models to data to generate predictions, rarely testing those predictions or learning from errors to improve both understanding and forecasting skill. With collaborators, LaDeau is developing approaches for iteratively cycling through a data-model-prediction-validation framework to advance ecological understanding.
This research has received a 2010 National Science Foundation (NSF) Research Coordination Grant to develop forecast models, a 2016 grant from NSF’s Macrosystems Biology Program, and a 2023 Gordon Research Conference on Prediction in Ecology.
Together with colleagues, LaDeau has been applying the data-model-prediction-validation framework to work toward forecasting algal blooms, which, if successful, would support fisheries management decision making and provide advanced notice of public safety hazards such as red tides. So far, this research has shown that water temperature is an important variable in forecasting cyanobacterial densities; it has also revealed new lines of research that could improve forecasting ability.
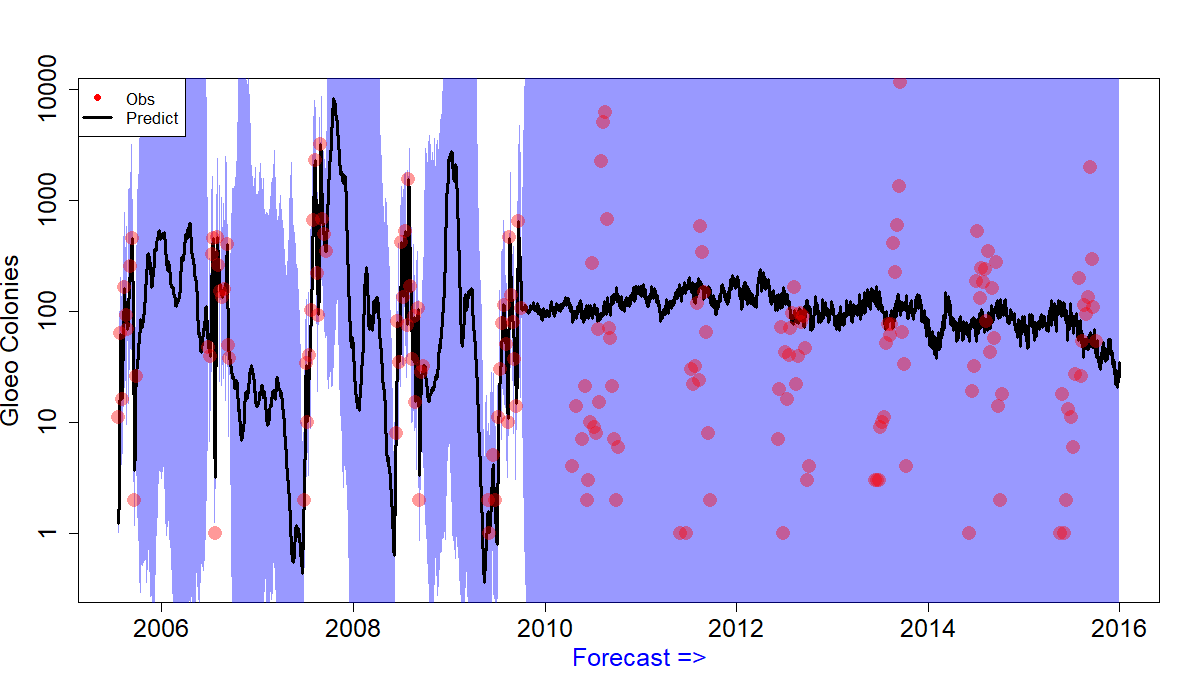
Leveraging data from Cary Institute’s long-term study of Lyme disease, LaDeau and colleagues are laying the foundation for real-time forecasting of tick populations. Their model integrates four stages of the tick life cycle — larvae, nymph, dormant nymph, and adult — together with weather variables and host population abundance to generate more accurate predictions. When they tested the model by making predictions in new locations, it performed well, pointing toward a future where seasonal Lyme disease risk can be forecasted. To refine the model and improve predictions, the team is continuing to investigate the relationship between climate, weather, and pathogen prevalence.
By applying ecological forecasting to ticks and other disease vectors, LaDeau hopes to refine predictions of disease risk and inform management practices to reduce those risks.
- Publications