In January, an algorithm created by the Canadian health startup BlueDot made headlines when it was revealed that it had warned of the coronavirus outbreak almost a week before the CDC or WHO. It's an impressive display of modeling, but once a disease has emerged, outbreak responders and public health officials are already behind the chains.
Barbara Han, a disease ecologist at the Cary Institute, is looking to put humanity on the front foot.
"What we want to be able to do is get ahead of that outbreak," Han says, "especially for diseases that end up causing large scale human losses" or destabilizing the global economy.
Using machine learning methods, Han is building models to predict where the pathogen that is going to cause the next major outbreak is most likely to come from. And more often than not, the next pandemic threat is going to come from animal to human diseases.
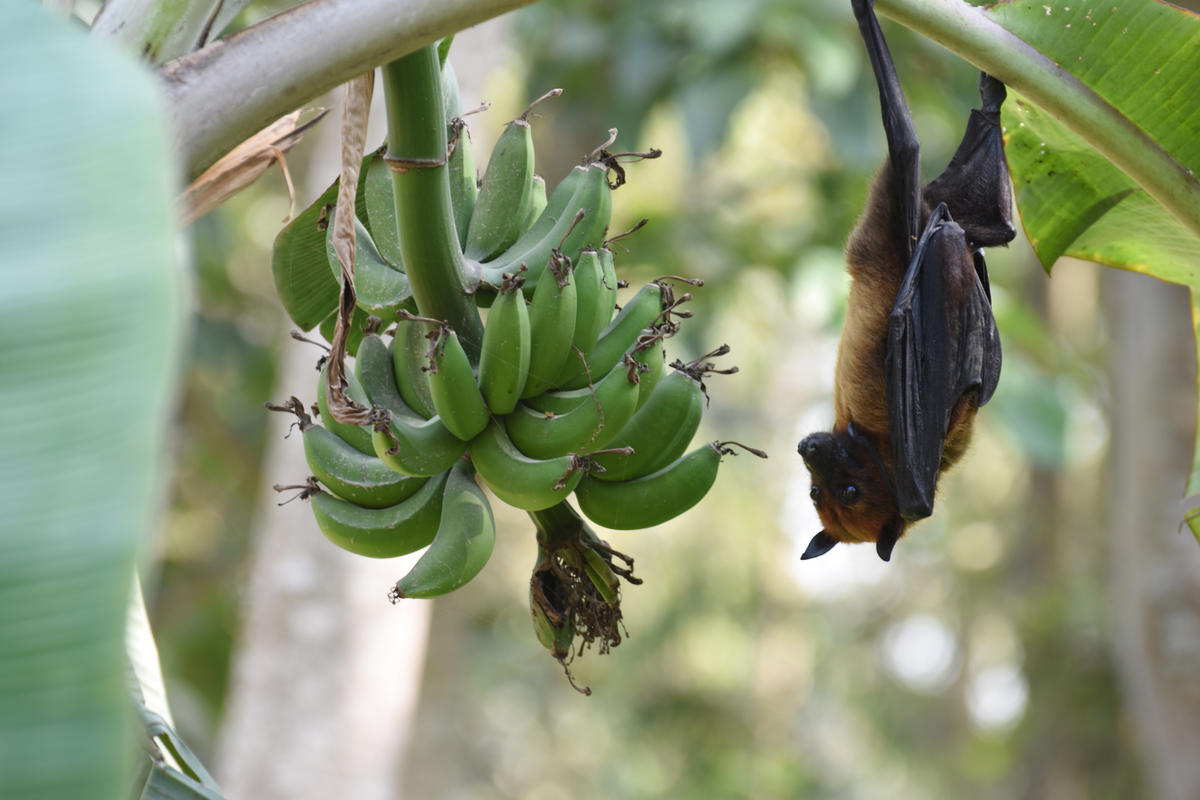
According to the CDC, 75% of emerging infectious diseases — pathogens we have not seen before, like the novel coronavirus, or are mutated enough that they may as well have never been seen — are zoonotic diseases. These animal-borne diseases sometimes mutate and make the jump into human beings, with potentially devastating consequences.
"It's really important to be pushing that envelope of prediction," Han says, "and making specific, testable predictions about species that have potentially high risk of transmitting pathogens to humans."
Finding a Zoonosis
Finding the animal hosts of these animal to human diseases is exceedingly difficult. Take bats, for example, which are noted reservoirs of viruses. Bats are a shockingly large cohort of mammals, second only to rodents — sheer variety is the first difficulty.
Once a certain species has been identified as a likely suspect, you then need to go collect lots of samples, often in remote and unpleasant locations, while avoiding getting sick yourself.
"It takes not only a physical toll but a mental toll," says Jason Kindrachuk, associate professor of viral pathogenesis at the University of Manitoba. "It's not as easy as you just go out, take a net, catch a bat, that's it."
There is often no way to know for sure what bodily fluid needs to be sampled — and to be avoided. A slip of the syringe could mean infection with the zoonosis. And there's also no guarantee that the bat you've wrangled, all leather, fangs, and fur, will have the virus; you need to catch the right one at the right time.
The whole ordeal is expensive, difficult, and absolutely necessary.
Predicting a zoonosis is hard enough when we know where to look — the domesticated pig and fowl populations of China, for example. But wild zoonotic diseases are much trickier, Kindrachuk says. "We don't know what they're going to do until they start to do it."
Finding the reservoirs of animal-borne diseases helps authorities potentially prevent outbreaks before they begin, says Biodun Ogunniyi, consultant epidemiologist and chief medical scientist at the Nigeria Centre for Disease Control (NCDC).
Even if an outbreak does happen, understanding how the zoonotic disease is transmitted can help to bring it under control faster. Lassa fever virus is carried by a certain type of rodent in Nigeria, the multimammate rat. Ensuring you eliminate or avoid that vector in populated areas will help both prevent an outbreak and stop one which has already happened.
Han has created models that use machine learning to predict the most likely reservoirs of zoonotic diseases, where they will be found, and how climate change and human activity may impact our chances of contracting them.
The decades' worth of animal data that has been painstakingly gathered by biologists — body sizes and physical characteristics, dietary habits, habitats and range, litters per year, lifespan — all helps paint a picture of the animals. Without this basic data, Han says, there is no way the model could predict the next zoonotic disease reservoir.
This gets combined with some molecular and cellular data that can help differentiate populations of the same species — rats in Norway are different from rats in Louisiana, Han says — as well as some coarse data on climate and human population density.
Together, the models can predict which bats are most likely to harbor zoonotic disease and which parts of the world should be focused on. By using her algorithm's results, researchers can best use their limited resources and improve the odds of the hunt.
"I think it would be very, very helpful," says Ogunniyi, "in not only controlling, but in preventing infection." In a country like Nigeria, where viral diseases like Lassa and yellow fever are endemic, being able to predict which animals, which populations, and which regions are likely to see zoonotic diseases emerge would help the NCDC stay ahead of the curve.
The emergence of novel zoonotic disease is essentially a given, and could be exacerbated as climate change disrupts the patterns of people and animals, bringing us closer into contact. A truly connected world, where a pathogen can pop on a plane and travel across the globe, may be especially at risk. If we are to weather the pandemics to come, we will need to be prepared — unfortunately, getting funding for an algorithm is not as easy as for a vaccine or antiviral.
As Han's models get tested more in the field, the success of the algorithms should continue to improve; every data point, whether it's good or bad, can hone the model and maximize the accuracy of the predictions.
"These models are what they are, given the data we have at hand," Han says. "There's no telling how powerful they could become given more data."